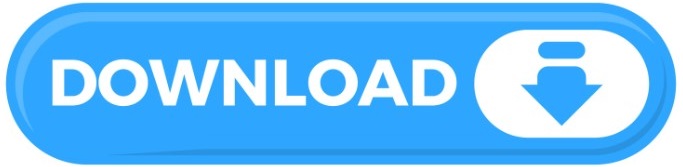


In this work, we present a method to detect missing annotations in hierarchical multi-label classification datasets. While these studies have shown great promise, the problem of annotating genes with functions remains an open challenge. Based on the interactions between genes (and gene products) extracted from the increasing genomic data, numerous studies have focused on the identification of associations between genes and functions. The availability of genomic data has grown exponentially in the last decade, mainly due to the development of new sequencing technologies. Finally, we provide guidelines to support this process when embarking on a hierarchical classification task, which will help optimize computational resources and predictive performance. The results show how different components of hierarchical data sets, such as variation coefficient and prediction by depth, can guide the choice of appropriate classification schemes. To fill this gap, here we have systematically contrasted the performance of 'Local per Level' and 'Local per Node' approaches with a 'Global' approach applied to two different hierarchical datasets: BioLip and CATH. These include 'Local' approaches considering the hierarchy, building models per level or node, and 'Global' hierarchical classification, using a flat classification approach. While some approaches to classify hierarchical data have been proposed, no guidelines regarding their utility, applicability and limitations have been explored or implemented. Biological data sets are often hierarchical in nature, with varying degrees of complexity, imposing different challenges to train, test and validate accurate and generalizable classification models. Given the complexity and scale of these databases, automatic data classification is often required. The rate of biological data generation has increased dramatically in recent years, which has driven the importance of databases as a resource to guide innovation and the generation of biological insights.
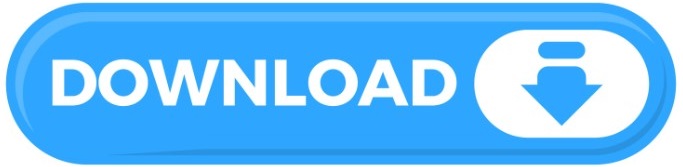